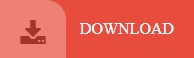
The SD hatplot marks a standard deviation above and below the mean, so the gray rectangle shows us the typical range of backpack weights that we calculated previously. So typical fifth and seventh graders are carrying between 7.0 and 21.4 pounds.

You should perhaps use a Bayesian estimate or Wilson score interval.The following histograms show the backpack weight carried by two groups of schoolchildren. Weighting by the inverse of the SEM is a common and sometimes optimal thing to do.
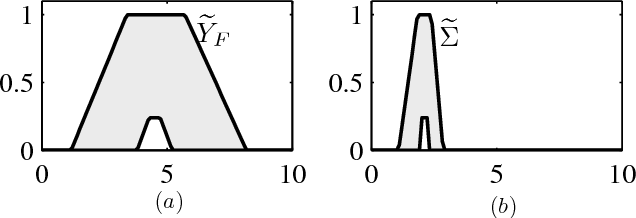
Taking percentages the way you are is going to make analysis tricky even if they're generated by a Bernoulli process, because if you get a score of 20 and 0, you have infinite percentage. You don't have an estimate for the weights, which I'm assuming you want to take to be proportional to reliability. Where $x^* = \sum w_i x_i / \sum w_i$ is the weighted mean.
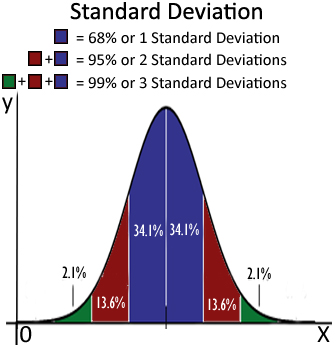
In any case, the formula for variance (from which you calculate standard deviation in the normal way) with "reliability" weights is (Actually, all of this is rubbish-you really need to use a more sophisticated model of the process that is generating these numbers! You apparently do not have something that spits out Normally-distributed numbers, so characterizing the system with the standard deviation is not the right thing to do.) Instead, you need to use the last method. You generate your data from frequencies, but it's not a simple matter of having 45 records of 3 and 15 records of 4 in your data set. In your case, it superficially looks like the weights are frequencies but they're not.

you are just trying to avoid adding up your whole sum), if the weights are in fact the variance of each measurement, or if they're just some external values you impose on your data. In particular, you will get different answers if the weights are frequencies (i.e. The key is to notice that it depends on what the weights mean. The formulae are available various places, including Wikipedia.
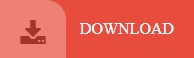